
Life Science Success
On this podcast, you'll find interviews with high-performing successful individuals in Life Sciences. On a weekly basis, we cover their proven methods, principles, strategies, and mindsets to implement new technologies that scale to meet the needs of people in our world.
Podcasting since 2020 • 298 episodes
Life Science Success
Latest Episodes
Inside the Brain: Stem Cell Treatments That Could Change Everything
In this episode of the Life Science Success Podcast my guest is Christopher Duma, a board-certified neurosurgeon who is pioneering innovative treatments for neurodegenerative diseases at Regeneration Biomedical, Inc.00:00 Introduction t...
•
53:42

Inside Pharma Packaging: Expert Strategies for Business Success
In this episode of the Life Science Success Podcast my guest is Frank Jäger, a Commercial Director at CCL Clinical and Managing Director of CCL Faubel, who brings extensive expertise in pharmaceutical labeling, sales, and innovative IoT applica...
•
37:26

Bridging Science & Business: Turning Research into Real-World Solutions
In this episode of the Life Science Success Podcast my guest is Oliver Loson, a Vice President of Business Operations at Holoclara, Inc. who brings a wealth of experience in translating scientific discoveries from academia to commercial applica...
•
52:02

AI in Pharma: A 30-Year Veteran's Roadmap to Digital Success
In this episode of the Life Science Success Podcast my guest is Sameer Lal, a highly respected pharma veteran and business leader with three decades of experience in advancing the life sciences industry through technological innovation. With hi...
•
36:10

Medical Tech Pioneer Janet Kwiatkowski: Strategies for Innovation & Growth
In this episode of the Life Science Success Podcast my guest is Janet Kwiatkowski, a proven executive leader with extensive experience in medical devices and in vitro diagnostic technologies.00:00 Introduction to Life Science Success Po...
•
26:01

Contributors
Podcasts we love
Check out these other fine podcasts recommended by us, not an algorithm.
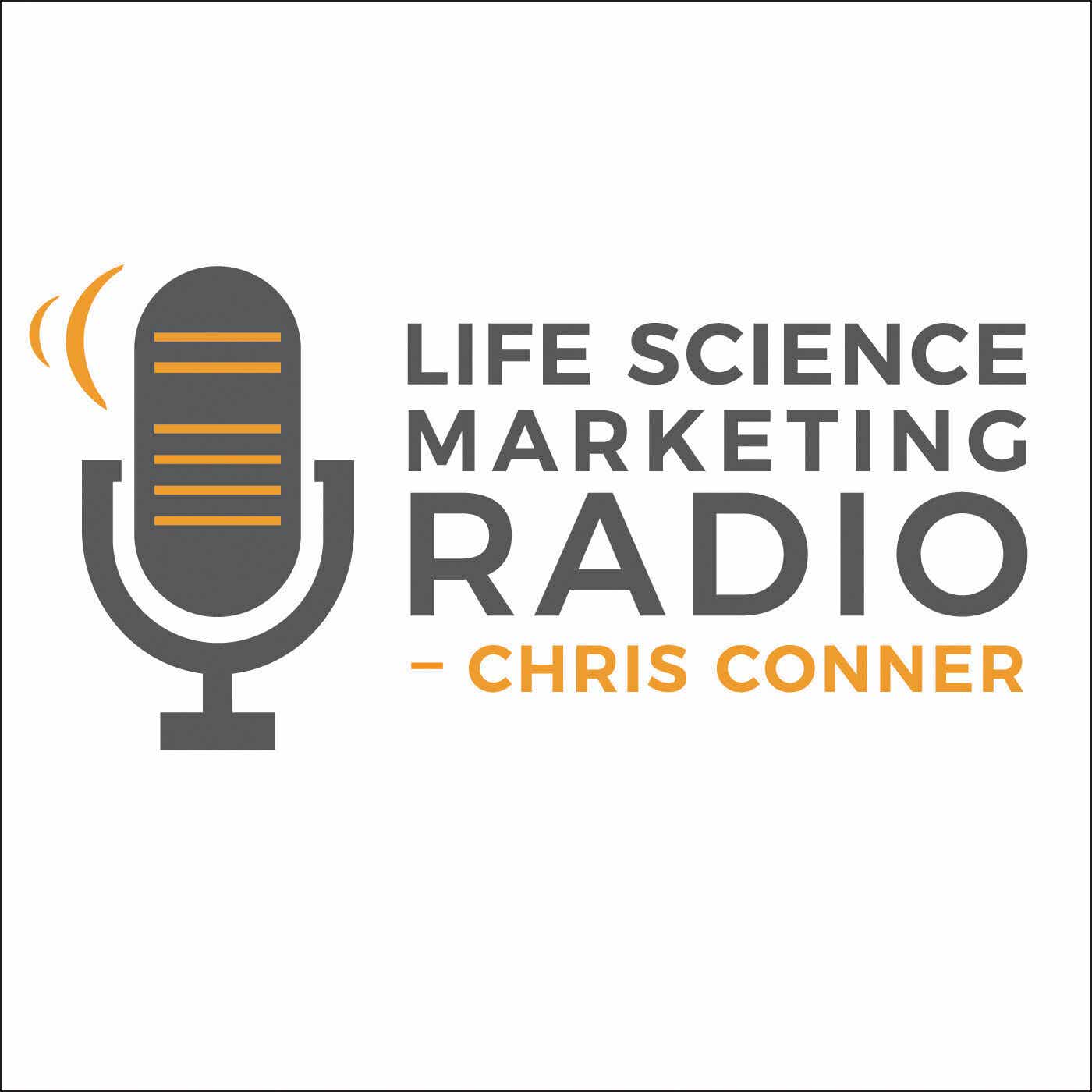
Life Science Marketing Radio
Chris Conner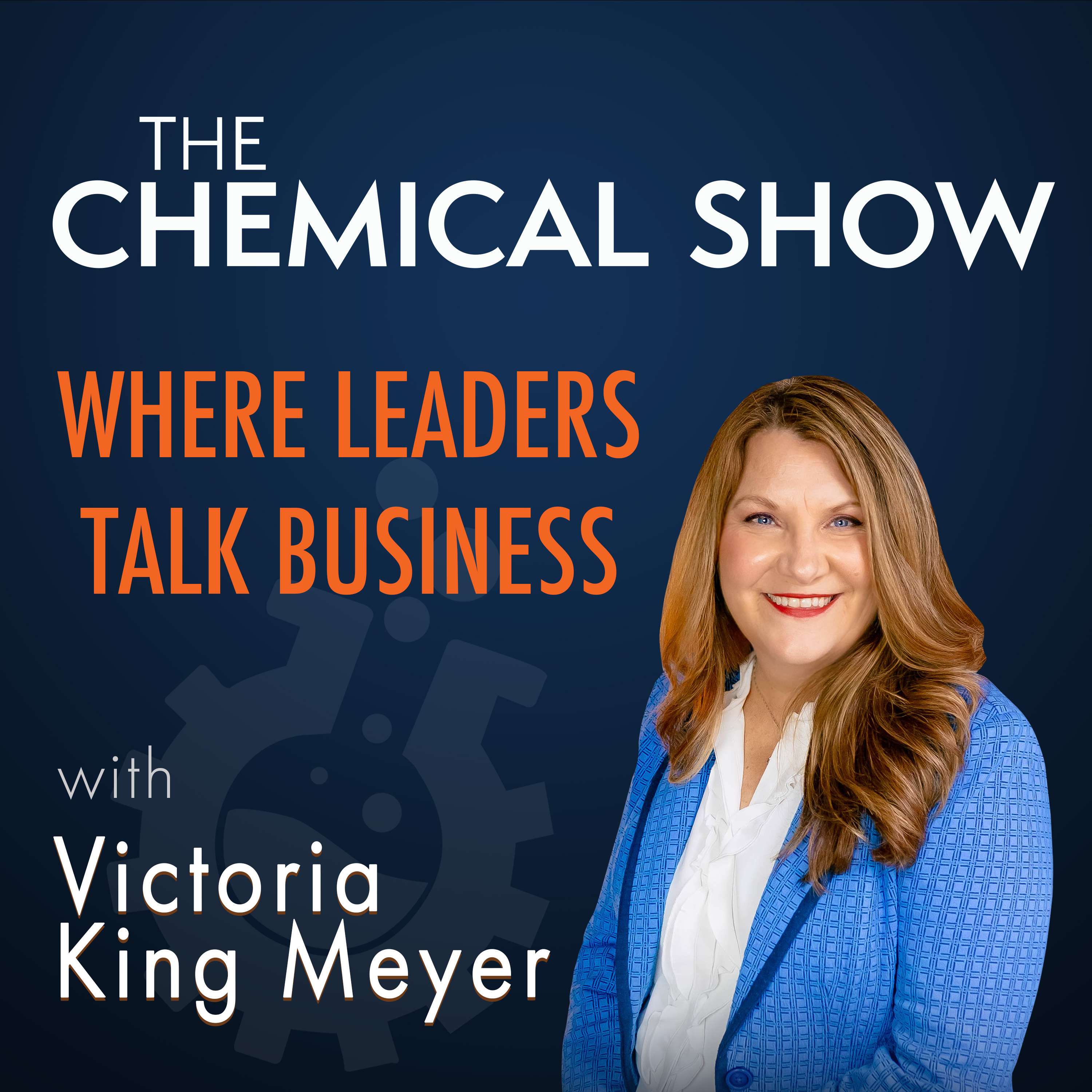